Tingkai Liu 刘廷恺
NeuroAI Researcher, Cold Spring Harbor Laboratory
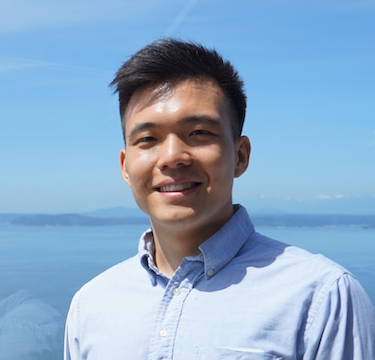
I am an independent researcher at Cold Spring Harbor Laboratory, part of the new NeuroAI research program, where I work on the intersection between artificial and biological intelligence.
From 2023 to 2024, I worked at ByteDance Inc. (AML), where I specialized in Large Language Model pre-/post-training and multi-modal foundation models.
I earned my MS/PhD in Electrical Engineering from Columbia University in December 2022, where I worked on theoretical and Computational Neuroscience. Prior to attending Columbia, I earned my BS degree at Rice University (Go Owls!).
news
Dec 3, 2024 | Our new work, ElastiFormer, is live on ArXiv! We propose a post-training technique that adapts pretrained Transformer models (e.g. LLM, ViT, VLM) into an elastic counterpart via self-distillation. We show that 20% to 50% compute saving could be achieved for different components of the transformer architecture, which could be further reduced by adding very low rank LoRA weights (rank 1) trained via the same distillation objective. |
---|---|
Aug 10, 2024 |
I’m presenting 2 of our papers at the 62nd Annual Meeting of the Association for Computational Linguistics (ACL) in Bangkok, Thailand from 08/11/2024 - 08/15/2024
|
research
My research covers a wide range of topics ranging from large language models pre-/post-training, multi-modal foundation models and computational neuroscience.-
2024
We introduce ElastiFormer, a post-training technique that self-distills any pretrained Transformer models into an elastic counterpart with variable inference time compute.
-
Annual Meeting of the Association for Computational Linguistics (ACL), 2024(Oral Presentation)
We introduce EVLGen, a one-staged single-loss framework based on Token Merging, for pre-training computationally intensive vision-language generative models using frozen pre-trained large language models.
-
Annual Meeting of the Association for Computational Linguistics (ACL), 2024
We present a novel task and human-annotated dataset for evaluating visual-language models’ ability to generate captions and summaries for real-world video clips.
-
arXiv, Oct 2023
We propose a loss-based LLM post-training data selection method method, which compresses Alpaca dataset by 16x while improving model performance on AlpacaEval.
-
arXiv, Oct 2023
We propose a heuristic greedy search algorithm based on step-level reward for improved LLM reasoning capabilities during inference. We also present a new automated way to generate massive amount of step-level reward signal for code generation tasks based on mutation testing.
-
arXiv, Oct 2023
We propose Video-Teller, a video-language foundation model with fine-grained modality alignment to enhance video-to-text generation tasks.
-
arXiv, Oct 2023
We introduce SimVLG, a one-staged single-loss framework based on Token Merging, for pre-training computationally intensive vision-language generative models using frozen pre-trained large language models.
-
US Patent US11674937B2, , Jun 2023
We developed a biomimetic method and apparatus for encoding odorants, which has been patented.
-
PLOS Computational Biology, Apr 2023
We provide theoretical and computational evidence for the functional logic of the Antennal Lobe as a robust odorant object identity recovery processor with ON-OFF event-based processing.
-
eLife, Feb 2021
We developed FlyBrainLab, an open-source computing platform that integrates 3D exploration and visualization of diverse datasets with interactive exploration of modeled executable brain circuits in Drosophila.
-
ICASSP 2020, May 2020
We present a bio-mimetic odorant encoding machine for sampling, reconstruction, and robust representation of odorant identity in the Drosophila olfactory system.
-
bioRxiv, Sep 2022
We demonstrate both theoretically and computationally that the Divisive Normalization Processor (DNP) is an invertible operator that can faithfully represent input information given sufficient output samples, with application to different sensory modalities.
-
bioRxiv, Sep 2022
We propose a feedback divisive normalization architecture of the Mushroom Body Calyx circuit in the Drosophila olfactory system for odorant demixing. We show that the biological network is highly optimized for processing odorant mixtures.
-
bioRxiv, Mar 2019
The Fruit Fly Brain Observatory is a platform that aims to bridge the gap between structural and functional data in neuroscience research on Drosophila. It provides tools for exploring and analyzing various types of fruit fly brain data, including connectome and functional imaging data.
-
FlyBrainLab: A Complete Programming Environment for Discovering the Functional Logic of the Fruit Fly BrainNeuroinformatics Assembly 2021, Apr 2021
-
FlyBrainLab: An Interactive Computing Platform for Exploring the Drosophila BrainPyData Global, Nov 2020
-
Comparative Study of Fruit Fly Brain Circuit Models with FlyBrainLabneuromatch 3.0, Oct 2020
-
FlyBrainLab: Interactive Computing in the Connectomic/Synaptomic EraJupytercon, Oct 2020
-
Functional Identification of the Odorant Transduction Process of Drosophila Olfactory Sensory NeuronsBMC Neuroscience 2020, 21(Suppl 1):P225, Jul 2020
-
Comparing Drosophila Neural Circuit Models with FlyBrainLabBMC Neuroscience 2020, 21(Suppl 1):P112, Jul 2020
-
The Geometry of Spatio-Temporal Odorant Mixture Encoding in the Drosophila Mushroom BodyBMC Neuroscience 2020, 21(Suppl 1):218, Jul 2020
-
Time-Dependent Simultaneous Configural and Elemental Odorant Mixture ProcessingCOSYNE*2020, Feb 2020
-
FlyBrainLab: An Interactive Computing Environment for the Fruit Fly BrainSociety of Neuroscience, Oct 2019
-
Divisive Normalization Circuits Faithfully Represent Visual, Olfactory and Auditory StimuliBMC Neuroscience 2019, 20 (Suppl 1): P353, Oct 2019
-
An Open-Source Model of the Fruit Fly Larval Mushroom BodyNeurobiology of Drosophila, Oct 2017